Program Committee
Area Chairs
International Program Committee
- Hojjat Adeli - USA
- Cesare Alippi - Italy
- Shun-ichi Amari - Japan
- Rafal A. Angryk - USA
- Robert Babuska - Netherlands
- James C. Bezdek - Australia
- Piero P. Bonissone - USA
- Bernadette Bouchon-Meunier - France
- Jinde Cao - China
- Juan Luis Castro - Spain
- Yen-Wei Chen - Japan
- Andrzej Cichocki - Japan
- Krzysztof Cios - USA
- Ian Cloete - Germany
- Oscar Cordón - Spain
- Bernard De Baets - Belgium
- Włodzisław Duch - Poland
- Meng Joo Er - Singapore
- Pablo Estevez - Chile
- David B. Fogel - USA
- Tom Gedeon - Australia
- Erol Gelenbe - United Kingdom
- Jerzy W. Grzymala-Busse - USA
- Hani Hagras - UK
- Saman Halgamuge - Australia
- Yoichi Hayashi - Japan
- Tim Hendtlass - Australia
- Francisco Herrera - Spain
- Kaoru Hirota - Japan
- Tingwen Huang - USA
- Hisao Ishibuchi - Japan
- Mo Jamshidi - USA
- Robert John - UK
- Janusz Kacprzyk - Poland
- Nikola Kasabov - New Zealand
- Okyay Kaynak - Turkey
- Vojislav Kecman - USA
- James M. Keller - USA
- Etienne Kerre - Belgium
- Frank Klawonn - Germany
- Robert Kozma - USA
- László Kóczy - Hungary
- Józef Korbicz - Poland
- Rudolf Kruse - German
- Adam Krzyzak - Canada
- Věra Kůrková - Czech Republic
- Soo-Young Lee - Korea
- Simon M. Lucas - UK
- Luis Magdalena - Spain
- Jerry M. Mendel - USA
- Radko Mesiar - Slovakia
- Zbigniew Michalewicz - Australia
- Javier Montero - Spain
- Eduard Montseny - Spain
- Kazumi Nakamatsu - Japan
- Detlef D. Nauck - Germany
- Ngoc Thanh Nguyen - Poland
- Erkki Oja - Finland
- Nikhil R. Pal - India
- Witold Pedrycz - Canada
- Leonid Perlovsky - USA
- Marios M. Polycarpou - Cyprus
- Danil Prokhorov - USA
- Vincenzo Piuri - Italy
- Sarunas Raudys - Lithuania
- Olga Rebrova - Russia
- Vladimir Red’ko - Russia
- Raúl Rojas - Germany
- Imre J. Rudas - Hungary
- Norihide Sano - Japan
- Rudy Setiono - Singapore
- Jennie Si - USA
- Peter Sincak - Slovakia
- Andrzej Skowron - Poland
- Roman Słowiński - Poland
- Pilar Sobrevilla - Spain
- Janusz Starzyk - USA
- Jerzy Stefanowski - Poland
- Vitomir Štruc - Slovenia
- Ron Sun - USA
- Johan Suykens - Belgium
- Ryszard Tadeusiewicz - Poland
- Hideyuki Takagi - Japan
- Dacheng Tao - Australia
- Vicenç Torra - Spain
- Burhan Turksen - Canada
- Shiro Usui - Japan
- Deliang Wang - USA
- Jun Wang - Hong Kong
- Lipo Wang - Singapore
- Paul Werbos - USA
- Bernard Widrow - USA
- Kay C. Wiese - Canada
- Bogdan M. Wilamowski - USA
- Donald C. Wunsch - USA
- Ronald R. Yager - USA
- Xin-She Yang - United Kingdom
- Gary Yen - USA
- Sławomir Zadrożny - Poland
- Jacek Zurada - USA
Invited Talks
Jan Chorowski "Representation learning for speech and handwriting"
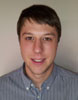
Head of AI at NavAlgo
Jan Chorowski is an Associate Professor at Faculty of Mathematics and Computer Science at the University of Wrocław and Head of AI at NavAlgo. He received his M.Sc. degree in electrical engineering from the Wrocław University of Technology, Poland and EE PhD from the University of Louisville, Kentucky in 2012. He has worked with several research teams, including Google Brain, Microsoft Research and Yoshua Bengio’s Lab at the University of Montreal. He has led a research topic during the JSALT 2019 workshop. His research interests are applications of neural networks to problems which are intuitive and easy for humans and difficult for machines, such as speech and natural language processing.
Learning representations of data in an unsupervised way is still an open problem of machine learning. We consider representations of speech and handwriting learned using autoencoders equipped with autoregressive decoders such as WeveNets or PixelCNNs. In those autoencoders, the encoder only needs to provide the little information needed to supplement all that can be inferred by the autoregressive decoder. This allows learning a representation able to capture high level semantic content from the signal, e.g. phoneme or character identities, while being invariant to confounding low level details in the signal such as the underlying pitch contour or background noise.
Presentation will include the design choices for the autoencoder, such as the bottleneck kind its hyperparameters impact the induced latent representation. Applications will be demonstrated to unsupervised acoustic unit discovery on the ZeroSpeech task. Discussion will cover how knowledge about the average unit duration can be enforced during training, as well as during inference on new data.
Włodzisław Duch "Brain-inspired cognitive computing"
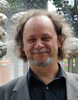
Google: W. Duch, CV: http://www.is.umk.pl/~duch/cv/cv.html
Brain are most flexible systems that can control any kind of bodies and extract useful information from many types of sensors. Understanding how brains accomplish such functions will provide inspiration for novel artificial intelligence algorithms. I will mention a few examples of mutual interplay between brain research and artificial intelligence algorithms. New methods of brain signal analysis that can discover "fingerprints" of brain activity are needed. Such research combined with brain-computer-brain interfaces will lead to new perspectives for treating mental disorders and improving human cognitive abilities. I will present some ideas that result from computational models of attractor networks, leading to hypothesis that can be verified using experimental techniques. 3 examples will be briefly presented: origin of autism/ADHD, formation of conspiracy theories and characterization of learning preferences. Progress depends on our ability to interpret brain signals, searching for traces of activation (fingerprints) of networks and brain regions. fMRI allows to assess changes in the activation of extensive brain networks as a result of cognitive load and working memory training. The great challenge is to develop practical methods, preferably based on EEG analyzed in real time, and use them for diagnosis of mental disorders, for neurofeedback or for control of neuromodulation methods that directly influence the structure of brain connections.
[1] Finc, K, Bonna, K, He, X, Lydon-Staley, D.M, Kühn, S, Duch, W, & Bassett, D. S. (2020). Dynamic reconfiguration of functional brain networks during working memory training. Nature Communications 11, 2435 (IF 11.8)
[2] Rykaczewski, K, Nikadon, J, Duch, W, Piotrowski, T. (2021). SupFunSim: spatial filtering toolbox for EEG. Neuroinformatics 19, 107–125
Janusz Kacprzyk "Advice giving and taking in decision aid, recommendation and support: the role of human judge and advisor"
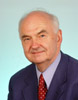
Full member, Polish Academy of Sciences
Member, Academia Europaea
Member, European Academy of Sciences and Arts
Member, European Academy of Sciences
Member, International Academy for Systems and Cybernetic Sciences (IASCYS)
Foreign member, Bulgarian Academy of Sciences
Foreign member, Finnish Society of Sciences and Letters
Foreign member, Royal Flemish Academy of Belgium for Sciences and the Arts (KVAB)
Foreign member, Spanish Royal Academy of Economic and Financial Sciences (RACEF)
Systems Research Institute, Polish Academy of Sciences
Ul. Newelska 6, 01-447 Warsaw, Poland
Email: kacprzyk@ibspan.waw.pl
Google: kacprzyk
Janusz Kacprzyk is Professor of Computer Science at the Systems Research Institute, Polish Academy of Sciences, WIT – Warsaw School of Information Technology, and Chongqing Three Gorges University, Wanzhou, Chinqgqung, China, and Professor of Automatic Control at PIAP – Industrial Institute of Automation and Measurements. He is Honorary Foreign Professor at the Department of Mathematics, Yli Normal University, Xinjiang, China. He is Full Member of the Polish Academy of Sciences, Member of Academia Europaea, European Academy of Sciences and Arts, European Academy of Sciences, Foreign Member of the: Bulgarian Academy of Sciences, Spanish Royal Academy of Economic and Financial Sciences (RACEF), Finnish Society of Sciences and Letters, and Flemish Royal Academy of Belgium of Sciences and the Arts (KVAB). He was awarded with 5 honorary doctorates. He is Fellow of IEEE, IET, IFSA, EurAI, IFIP and SMIA.
His main research interests include the use of modern computation computational and artificial intelligence tools, notably fuzzy logic, in systems science, decision making, optimization, control, data analysis and data mining, with applications in mobile robotics, systems modeling, ICT etc.
He authored 7 books, (co)edited more than 150 volumes, (co)authored more than 650 papers, including ca. 100 in journals indexed by the WoS. His bibliographic data are: Google Scholar: citations: 30596; h-index: 77, Scopus: citations: citations: 9111; h-index: 41, Web of Science: citations: 7228; h-index: 37. He is listed in 2020 ”World’s 2% Top Scientists” by Stanford University, Elsevier (Scopus) and ScieTech Strategies and published in PLOS Biology Journal.
He is the editor in chief of 7 book series at Springer, and of 2 journals, and is on the editorial boards of ca. 40 journals.. He is President of the Polish Operational and Systems Research Society and Past President of International Fuzzy Systems Association.
A growing complexity of technological, economic and social settings in which decision making processes occur, combined with their human centricity, time criticality, huge possible consequences of good and bad choices and actions, to just name a few, call for an effective and efficient approach to their solution. For the time being, and for a foreseeable future, its seems that for non-trivial problems, in which the human being is and will be a key element, the best paradigm should be not the „full automation”, the so called automated decision making (ADM) but rather the so called quasi-automated decision making (Quasi-ADM) which boils down to a synergistic combination of human and computer capablities, notably by a combination of a remarkable human ability to deal with delicate and sophisticated aspects and a sheer number crunching ability of the computer.
We are concerned with broadly perceived decision making problems that use optimization as a tool to formulate and solve problems, maybe also using metaheuristics. We consider first what can make the problem of an optimization type difficult to solve. We present the idea of the so-called decision aid as an effective and efficient solution paradigm. Due to the complexity and the problem and solution tools, we assume that the decision maker (judge), who is an expert in his/her field but not necessarily in the solution methods, commisions an analyst (advisor), who is an expert in the solution methods but not necessarily in the problem concerned. The judge makes the final judgment (or decision), i.e. has the decision making power, while the advisor just provides advice, information, or suggestions.
First, we analyze the division of work in such a setting and show its advantages and disadvantages. We also mention that it implies a change in the human – computer interactive problem solving, from the old command based to a new advice based one. Since there are 3 stakeholder (client, analyst, and the pair „client-analyst”), we are concerned with complex relations between them, notably related to the advice giving (by the advisor) and advice taking (by the judge). i.e. advice utilization. In particular, we discuss advice discounting that makes the extent of advice utilization lower. We briefly mention some approaches in this respect, notably those attributing advice discounting by the judge to not knowing the advisor’s internal reasons for his/her opinion, using own opinions (by the judge) as a starting point and using the advice just as their adjustment, a human deficiency (of the judge) considering himself/herself to be superior to the „subordinate” advisor, and also some other issues..
Then, we mention similar settings while using the recommenders (recommendation systems) and decision support systems as a different, also highly effective and efficient way of solving complex problems.
Nikhil R. Pal "Artificial Intelligence: "Winters", "Booms", and what we might be missing!"
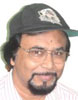
Head, Center for Artificial intelligence and Machine Learning
Indian Statistical Institute, Calcutta, India
Nikhil R. Pal is a Professor in the Electronics and Communication Sciences Unit and is the Head of the Center for Artificial Intelligence and Machine Learning of the Indian Statistical Institute. His current research interest includes brain science, computational intelligence, machine learning and data mining.
He was the Editor-in-Chief of the IEEE Transactions on Fuzzy Systems for the period January 2005-December 2010. He has served/been serving on the editorial /advisory board/ steering committee of several journals including the International Journal of Approximate Reasoning, Applied Soft Computing, International Journal of Neural Systems, Fuzzy Sets and Systems, IEEE Transactions on Fuzzy Systems and the IEEE Transactions on Cybernetics.
He is a recipient of the 2015 IEEE Computational Intelligence Society (CIS) Fuzzy Systems Pioneer Award, He has given many plenary/keynote speeches in different premier international conferences in the area of computational intelligence. He has served as the General Chair, Program Chair, and co-Program chair of several conferences. He was a Distinguished Lecturer of the IEEE CIS (2010-2012, 2016-2018.) and was a member of the Administrative Committee of the IEEE CIS (2010-2012). He has served as the Vice-President for Publications of the IEEE CIS (2013-2016). He has served as the President of the IEEE CIS (2018-2019).
He is a Fellow of the National Academy of Sciences, India, Indian National Academy of Engineering, Indian National Science Academy, International Fuzzy Systems Association (IFSA), The World Academy of Sciences, and a Fellow of the IEEE, USA. ( www.isical.ac.in/~nikhil)
In this talk I shall briefly go through the history of evolution of AI – how AI has sailed through the “ups and downs” and has come to the present state. In the recent past, we have witnessed numerous fantastic success stories of AI systems, often beating human performance, and this has caused our expectation from AI to skyrocket. In many cases, neural networks, in particular deep neural networks, are the main pillars of such systems. But are these systems comprehensible and/or biologically plausible? In most cases, they are not! It seems, implicitly we have started believing in philosophies like "bigger the better" (bigger data sets or massive architecture with millions of free parameters) and "data say all". Such approaches have been proved to be useful but raise some concerns too! In my view, comprehensibility of a system depends, at least, on the following: simplicity, transparency, explainability, trustworthiness, and in some cases the biological plausibility of such systems. Ideally, we should strive for realizing all these attributes in any AI system, but this is very difficult. I shall discuss some of these important issues where we need to pay more attention and then illustrate how one or two of these issues can be addressed (to some extent) borrowing knowledge from biological systems- some of our preliminary attempts.
Contact - ICAISC 2021 Organizing Committee
Databases and Internet Submission
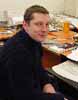
Finance Chair
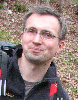
Organizing Committee Office
Institute of Computational Intelligence Częstochowa University of Technology al. Armii Krajowej 36 42-200 Częstochowa, Poland Tel: +48 (34) 3250546 Fax: +48 (34) 3250546 E-mail: icaisc@pcz.pl ICAISC 2021 Web page: http://icaisc.euThe Conference is organized by:
in cooperation with